Tutorials speakers
The totorials dedicated to newcomers into the machine learning world will be given by:
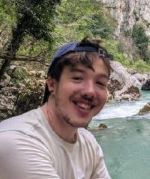
Anatole Moureaux UCLouvain, Belgium
|
|
Surfing the AI wave: foundations, current feats, and future challenges
Since their first developments 80 years ago, the different techniques commonly referred to as 'Artificial Intelligence' have not stopped evolving. The field has known a remarkable diversification, leading to an ever-increasing number of applications in industry, research, health, and everyday life with impressive success. The development of high-performance computers with increased capabilities and the creation of new architectures and paradimgs has made AI more compelling than ever. Recently, the emergence of ChatGPT has brought AI to the forefront of the general public's attention. But what exactly is all that fuss about? Which techniques lie underneath the AI umbrella? What's under the hood of these famous neural networks, how do they work, and how can we use them? We will try to answer all these questions at the light of the last developments in the discipline. We will also have a look to a few of the most impressive feats of AI throughout history, as well as the future challenges ahead.
|
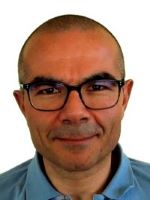
Prof. Gian-Marco Rignanese UCLouvain, Belgium
|
|
Materials process and property predictions from limited and multi-fidelity datasets
The available materials data are often rather limited. Unfortunately, typical machine-learning approaches generally require large amounts of data to make accurate predictions. To tackle this limitation, I will introduce MODNet, an all-round framework which relies on a feedforward neural network, the selection of physically meaningful features, and when applicable, joint-learning. Next to being faster in terms of training time, this approach is shown to outperform current graph-network models on small datasets. Furthermore, materials data often present various levels of accuracy, with typically much less high- than low-fidelity data. I will present an approach to extract as much information as possible from all available data by improving the quality of the data through denoising. Finally, I will discuss how active learning can be used to optimally perform data acquisition.
|
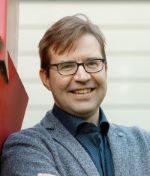
Prof. Stefan Sandfeld Forschungszentrum Jülich, Germany
|
|
Introduction to Machine Learning and Data Science
This tutorial gives an introduction to the most important concepts and tools of machine learning and data science with particular emphasize on materials science. It starts with an overview of the most important notions and elucidates them based on small but concrete examples. The most important machine learning approaches and models are introduced, ranging from approaches for regression, classification, clustering. Last but not least, deep learning methods discussed which are particularly important for microscopy images.
|
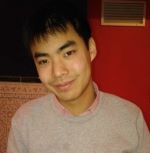
Dr. Julien Lam Chargé de recherches CNRS, Université de Lille
|
|
Atomistic simulations using machine-learning interaction potentials
Atomistic simulations have played a crucial role in the discovery of novel materials and the understanding of their specific properties. In this context, quantum calculations, including ab initio and density functional theory are the most accurate for calculating equilibrium properties and provide quantitative results comparable to experiments. In the meantime, empirical interaction potentials have also been employed to perform larger scale simulations yet with significantly lower accuracy, when compared to quantum calculations. In the past couple decades, machine-learning have been proposed as a way to bridge the gap between quantum accurate calculations and fast empirical modeling.
In this tutorial, we will give a short review on machine-learning interaction potentials describing the theoretical background before showing examples targeted to nanomaterial modeling.
|
|